A crisp understanding of how it is reshaping the AI realm!!
Table of Contents
Introduction
One of the most revolutionary developments in recent years in the quickly changing field of technology is Artificial intelligence (AI) and Gen AI (GenAI) Applications. This potent collection of AI models has set off a revolution, opening up previously unheard-of possibilities that are revolutionizing entire industries, resolving challenging issues, and dramatically increasing human productivity.
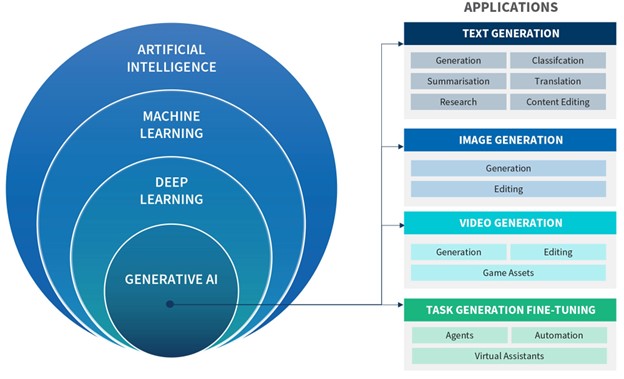
GenAI’s ability to produce text, graphics, and even audio that resembles human speech in answer to open-ended questions is fundamental to its significance. GenAI models, such as GPT-3, DALL-E, and Stable Diffusion, have shown a surprising breadth of capabilities, smoothly adapting to a wide range of AI Applications, in contrast to classic AI systems that excel at restricted, well-defined tasks.
This post will examine the real-world issues that GenAI is ideally suited to resolve and consider how it will elevate the tech Realm.
GAN
[Technical Overview of GANs and Their Real-Life Applications]
As we saw in the last article, Generative Adversarial Networks (GANs) are a revolutionary deep-learning architecture that has transformed the field of generative modeling. At the core of a GAN are two neural networks, the generator and the discriminator, that engage in an adversarial game to produce realistic, synthetic data that can be used for several different problem statements.
The generator network is tasked with creating indistinguishable samples from accurate data. In contrast, the discriminator network aims to identify the generated samples as fake so that the input data can be read better and produce real-time data. This continuous interplay between the two networks drives the generator to improve its ability to generate highly credible, almost natural, and dependable samples, effectively mimicking the underlying data distribution.
GANs have found numerous real-life AI applications across diverse domains. They have been used in computer vision to generate photorealistic images, enable high-quality image-to-image translation, and enhance low-resolution images, which can now be further analyzed. In natural language processing, GANs have been leveraged for text generation, style transfer, and dialogue systems, i.e., changes in Syntax or semantics.
Beyond media generation, GANs have also been applied to financial modeling, verified by the Time-Series analysis of the preexisting data, medical image analysis, and even the creation of synthetic training data for machine learning models, which rules out the need for data augmentation for data-scarce ML models. As the capabilities of GANs continue to evolve, their impact on various industries is poised to expand exponentially, transforming how we interact with and leverage generative AI technologies.
RAG
Retrieval-augmented generation (RAG) is a novel AI architecture that combines the power of language models with the knowledge retrieval capabilities of dense retrieval systems. This hybrid approach generates more informed, context-aware, and factually consistent outputs.
A RAG model’s core is a language model trained to generate text and a retrieval module that can dynamically access and incorporate relevant information from a knowledge base during the generation process. This enables the model to draw upon external knowledge beyond what is contained in its training data to produce more coherent and informative responses. This would mean you would have highly specific chatbots that would help in critical decision-making, such as a Neurosurgical Chatbot to help out neurosurgeries.
The real-life AI Applications of RAG are vast and diverse. RAG models can provide more comprehensive and accurate answers to question-answering by retrieving relevant facts and details from a knowledge base. This can significantly enhance the capabilities of virtual assistants and chatbots, making them better equipped to handle complex queries.
In content creation, RAG can generate high-quality, fact-checked articles, reports, and summaries by seamlessly integrating relevant information from authoritative sources or other GEN AI tools. This can be particularly valuable in journalism, academic writing, and scientific research.
Furthermore, RAG’s ability to combine language understanding with knowledge retrieval can be applied to tasks like task-oriented dialogue, personalized recommendation systems, and creative writing. The model can draw upon a diverse knowledge base to generate unique and contextually relevant outputs.
As AI continues to evolve, the adoption of RAG and similar hybrid architectures is expected to grow, unlocking new possibilities for more intelligent, informative, and engaging AI applications across various industries and use cases.
You can learn all about RAGs and how to build them using Langchain’s official documentation, the link to which is given below.
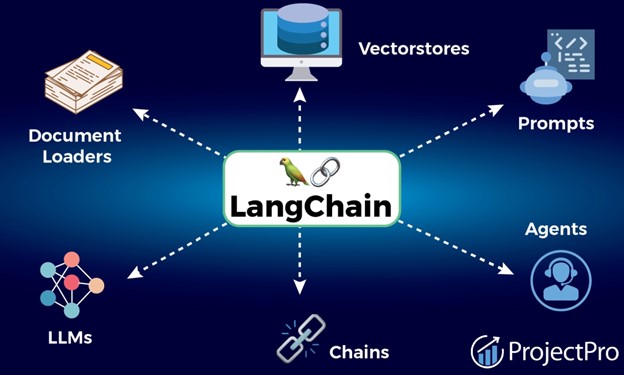
Picture_Credit : https://images.app.goo.gl/PF7Z7gAV5i8XPgAcA
Other Problem Statements:
- Language barriers in global business Application: Real-time GenAI-powered translation systems killing communication barriers in multinational corporations, enabling more efficient collaboration and expanding market reach.
- Climate model complexity and computational demands Application: GenAI models are being developed to emulate complex climate models, allowing faster and more efficient climate simulations to support policy decisions and research.
- Supply chain disruptions Application: GenAI models analyze complex supply chain data and generate predictive insights, helping businesses anticipate and mitigate potential disruptions. You can check out the Ernst and Young documentation for the same.
https://www.ey.com/en_gl/insights/supply-chain/how-generative-ai-in-supply-chain -can-drive-value
As we have shown, generative artificial intelligence (GenAI) has a wide range of transformational AI applications that solve various real-time difficulties across industries. GenAI has become a disruptive technology transforming how we work, communicate, and solve problems. It can speed up content creation, improve customer experiences, spur innovation, and close the digital gap.
However, as GenAI’s impact increases, we must prioritize creating morally sound legal frameworks to guarantee that these potent tools are used to advance humankind.
Exemplary GenAI applications
1. LUCY.AI
Lucy is a modern search tool that allows companies to access their server data with a straightforward natural language question. It works inside a client’s chat system, such as Microsoft Teams or Slack. The founders of Lucy call it an “answer engine” rather than a search engine.
“We make a promise that you can find information you did not even know existed in your company,” says Steve Frederickson, chief product officer of Lucy.ai. “Lucy is the fulfillment of that promise.”
The company’s first product used an AI platform to find answers. However, as demand for Lucy and customer data stores grew, the amount of data “she” had to search increased beyond her ability.
It was time to build a new AI infrastructure to handle the proliferation of data she was swimming in. The company worked with Microsoft Azure services, tapping a suite of products to build a secure system that could search any kind of data, including video, to find, in seconds, the answer to a user’s question about their data.
The newly rebuilt Lucy can find that answer — in videos, PDFs, PowerPoint presentations, and more — in seconds in response to natural language questions.
Results
- Newly built AI infrastructure
- Tool adopted by 100% of customers who try the new video indexer
- Tool pinpointed precise moment in a video that answers a user’s query
The official LUCY documentation is: https://www.lucy.ai/
2. Booking.com
Booking.com wanted to tap AI to make it easier for customers to find a travel itinerary. The company has a history of data-driven innovation and vast stores of data—content, reviews, and booking details—that could help. However, according to a case study, that data was complex for customers to filter.
The company worked with Amazon Bedrock to build a tool to quickly sort through the data to respond to customers’ natural language questions about travel. The company already used AWS for data storage and machine learning (ML).
“Generative AI is on everyone’s agenda right now,” says Thomas Davey, VP of big data and machine learning at Booking.com. “We can pick the right language models and fine-tune them with Booking.com data to deliver destination and accommodation recommendations that are tailored and relevant.”
The company built an AI Trip Planner that allows customers to have an in-app conversation with an AI about where they want to go and what they want to do when they arrive. It strips out the customer’s data and then taps Booking.com’s vast reviews and travel information data to make recommendations. It creates a carousel of options customers can flip through and book within the app.
Results
- Turned decades of user reviews into instant recommendations
- Helped customers find itinerary ideas they never would have thought of
- Quickly stripped personal data from in-app conversations to protect user privacy. The official AI documentation: https://news.booking.com/how-bookingcoms-ai-trip-planner-can-inspire-travelers-to-p lan-that-last-minute-summer-vacation/
3. Minijob Zentrale
Minijob-Zentrale (MJZ) is a German agency that gathers and reports on data and news about part-time jobs. A team of editors publishes articles intended to engage and inform an audience of interested parties. The team often reports on complex regulatory issues and news. According to a case study, they found it challenging to develop story ideas and write articles on this topic that were both interesting and accurate.
The team worked with IBM and its watsonx.ai. The generative AI analyzed MJZ’s workflows and content to create an AI editorial assistant, Mini, to help them with the work. Mini simplified texts, generated ideas, and helped adapt stories and data for the target reader.
With Mini on the team, lifting some of the workload from the editors, the team could write and publish more articles faster while maintaining accuracy and creative control.
“With this tool,” says Madeline Scholz of Minijob-Zentrale, “we will need only a quarter of the time compared to before to plan, write, and publish an article.”
The AI also helped editors better understand and empathize with their readers, so the content they write and publish is on target.
Results
- 75% less time to publish articles
- Simplified complex editorial texts
- Learned about the audience
Official IBM Documentation: https://www.ibm.com/case-studies/minijob-zentrale
Looking for more on AI? Here’s another article you’ll find insightful! – Artificial Intelligence in Healthcare – a New Era of Medicine [https://embedtalk.com/artificial-intelligence-in-healthcare-a-new-era-of-medicine/]
References
- https://www.analyticsinsight.net/artificial-intelligence/10-complex-problems-that-generative-ai-has-solved-in-2022
- https://www.lexisnexis.com/blogs/ae/b/data-as-a-service/posts/challenges-of-generative-ai?srsltid=AfmBOor-IxD5gTg7gujxvjuIERBRHzF
- https://tll.mit.edu/rethinking-your-problem-sets-in-the-world-of-generative-ai/
Leave feedback about this